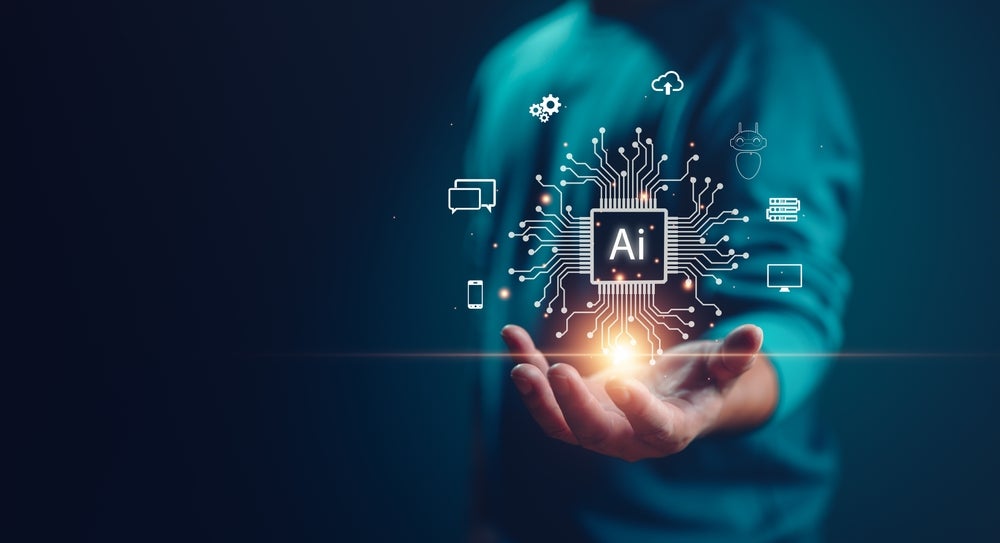
When AI entered the mainstream, it instilled a sense of panic in many people. It was seen as a threat that would replace humans across the working world. Given the speed at which AI is advancing, and the emergence of technologies such as ChatGPT, this fear may seem reasonable.
But over time, we’ve started to see AI as a helpful tool, not a replacement for humans. And just like any other tool, it can be used for good or bad. As a result, the real threat AI poses is not mass redundancies. It’s the pace at which malicious actors have exploited it to commit crime.
To respond effectively, financial institutions need to fight fire with fire, using AI to limit the ability of lawbreakers to get away with their crimes. In doing so, it’s essential to balance the analytical prowess of AI and the nuanced understanding of humans. So, what does this collaboration look like?
The tools of the modern age
In the fight back against AI-enabled fraud, counter measures using defensive AI and data analytics have become invaluable. The sheer volume of transaction data can easily overwhelm human analysts attempting to deal with threats, making it nearly impossible for humans to thoroughly review and detect suspicious activity. This leaves room for potential crimes to go undetected.
AI, through machine learning algorithms and advanced analytics, can quickly and accurately analyse large amounts of data, identifying patterns and anomalies that indicate fraudulent activity. This not only helps prevent financial crimes but also saves time and resources for financial institutions.
However, investigations are not a solo act. Humans play a crucial role in reviewing these AI-detected cases, making critical decisions based on AI recommendations. This symbiotic arrangement creates a more efficient and effective approach in combating financial crime.
How well do you really know your competitors?
Access the most comprehensive Company Profiles on the market, powered by GlobalData. Save hours of research. Gain competitive edge.
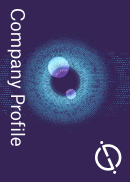
Thank you!
Your download email will arrive shortly
Not ready to buy yet? Download a free sample
We are confident about the unique quality of our Company Profiles. However, we want you to make the most beneficial decision for your business, so we offer a free sample that you can download by submitting the below form
By GlobalDataFor instance, an AI system may flag a potential fraudulent transaction, but a human is needed to review and ultimately decide on the best course of action. This collaborative approach can help minimise false positives and ensure a balanced system.
The recent AI boom has highlighted that a wider understanding of the world and its current affairs is crucial. Financial crime is not just about numbers and data, but also involves complex reasoning and nuance in decision making. Humans can consider various factors and make judgments based on their knowledge and experience, which AI may not be able to do.
Additionally, AI operates solely on the data on which it has been trained. This can sometimes be incomplete or biased. This highlights the importance of human involvement, as they can provide a critical perspective that AI may not be able to capture.
The challenge of staying objective
The importance of human involvement in investigations cannot be understated, but it has its challenges. Supervised machine learning models are commonly used in fraud and money laundering detection. These algorithms learn from human labelled or annotated historical data. They connect transaction attributes with investigator decisions to identify potentially suspicious activities.
Supervised algorithms work as part of a feedback loop. It’s like a dance, where AI makes a move, the investigator responds, and the AI adjusts its next move based on that response. However, human decision-making can introduce bias or errors, which could unwittingly skew AI recommendations. The choices humans make, such as accepting or rejecting transactions, influence how AI learns.
One solution to reduce model bias is to adopt a combination of supervised and unsupervised methods. Unsupervised learning requires the model to find hidden patterns in unlabelled data. This can be a more objective and unbiased approach. By using different detection techniques investigators can get a broader view of the activities they’re monitoring.
In addition, it’s critical to actively and regularly monitor and evaluate the AI system’s performance. This ensures that the AI’s recommendations stay accurate and fair, preventing unintentional biases caused by human decisions from affecting the results.
Changing the game
The advent of Generative AI, particularly large language models (LLMs), is set to transform how investigators interact with AI. OpenAI’s ChatGPT and similar tools are already finding applications across various fields and it’s only a matter of time before they become widely used in financial crime investigations.
LLMs offer two stand out capabilities that could be game-changers. First, they excel at handling unstructured data, allowing them to easily decipher information from diverse data formats. Second, they demonstrate advanced reasoning abilities, enabling them to perform complex tasks based on user instructions.
All this means that LLMs can automate tasks that were once thought to be too complex for AI. This includes querying databases, extracting information from documents, interpreting, aggregating, and contextualising data. These capabilities help investigators by providing real-time information and analysis of data from different sources.
For example, if a suspicious transaction is flagged, an AI chatbot can quickly gather relevant data from databases, AI models, and documents. It then presents this information to the investigator in a summarised, focused format, allowing them to make informed decisions promptly.
It is easy to envision a future where investigations evolve into a conversation between AI and humans. In this future, the AI acts as an assistant gathering key information to the investigator, who ultimately makes the final decision.
Collaboration is the best defence
When it comes to combatting financial crime, it essential for humans and AI systems to be working on the same page. To stay one step ahead of AI-enabled fraudsters, it is important to understand the ways in which human decision-making can shape AI recommendations.
There needs to be a continuous monitoring and evaluation of these systems to ensure that these AI systems continue to be effective. Even with the potential for human error, the benefits of enlisting AI to create a collaborative approach are exponential.
Daoud Abdel Hadi is Lead Data Scientist at Eastnets and Seun Sotuminu is a Data Scientist, PDM, Eastnets