Even with alternative credit scoring, few lenders provide meaningful transparency around how they score would-be borrowers, nor the pricing categories underpinning those scores. This suggests a gap in the market for radical transparency explicitly tied to credit scoring, as opposed to transparency around ratings and reviews, ESG compliant lending/investing, or fees. Banks’ digital money management tools could underpin this, providing help to improve consumers’ financial situation but in a way that directly correlates with improved chances of accessing credit from that same lender.
Regulatory Trends
Listed below are the key regulatory trends impacting the alternative credit scoring theme, as identified by GlobalData.
How well do you really know your competitors?
Access the most comprehensive Company Profiles on the market, powered by GlobalData. Save hours of research. Gain competitive edge.
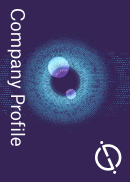
Thank you!
Your download email will arrive shortly
Not ready to buy yet? Download a free sample
We are confident about the unique quality of our Company Profiles. However, we want you to make the most beneficial decision for your business, so we offer a free sample that you can download by submitting the below form
By GlobalDataFocus on financial inclusion and fair treatment
Regulators worldwide are fixated on driving fair, transparent lending decisions. Housed within the Consumer Financial Protection Bureau of the US, all federal lenders would be required to incorporate the federal credit agency’s scoring, including for programmes such as federal home lending, PLUS loans, and other loans that are guaranteed by the US government. This is an unprecedented move the will attack the root causes of poverty and inequality, which other regulators will be watching closely.
Growing awareness of alternative data gone too far
Continued digitisation – through the adoption of platforms that deliver early customer insights – can provide a pathway to implement stringent affordability and income verification assessments, while identifying financially vulnerable customers and abiding by regulatory requirements. However, it is also true alternative data can go too far. Case in point: China’s public sector social credit system uses 1,000 individual data points.
Scores can be improved by donating blood or money, engaging in charity work, praising the government on social media, and helping the poor. Chinese citizens can lose points by not visiting their aging parents regularly, cheating in online games, insincere apologies for crimes committed, and spreading rumours on the internet.
Data-driven credit scoring confers market power on data-driven businesses that may cost consumers
Given their scale and technology, big techs have the ability to collect massive amounts of data at near-zero cost, creating “data-opolies.” Because a key part of picking winners in algorithms is an ability to learn over time, first-mover advantage can make it difficult for second-movers to catch up – not just near term, but ever.
Once their dominant position in data is established, big techs can engage in price discrimination and extract rents. They may use their data not only to assess a potential borrower’s creditworthiness, but also to identify the highest rate the borrower would be willing to pay for a loan or the highest premium a client would pay for insurance.
Reputational and regulatory risks of alternative credit scoring for incumbent banks
Endorsing or promoting new technologies and approaches without testing reliability and consistency could harm reputations, which is part of the reason incumbent banks evolve practices more slowly than new entrants. It’s also true that when banks try new things – like new credit models – regulators require them to keep disproportionate amounts of equity capital in reserve, and while their debt capital (i.e. deposits) is cheap, bank equity capital is expensive.
Open banking
Open banking makes it easier for a vast array of start-ups to obtain more granular data from alternative and traditional data sources. For big techs, open banking enables them to combine their own data with other geolocation and behavioural data to measure creditworthiness, with each new application measured helping improve the accuracy and predictability of the algorithm further, which makes it harder for other players to catch up over time.
Balance between algorithmic predictive power and transparency
Marketplace lenders’ business models are based on proprietary algorithms labelled as trade secrets, which protect the exact scoring methods for all credit scores. Frequently, credit rating companies justify the secrecy as a means to keep competitors from learning how their systems are built and operated, and to prevent scored individuals from deceiving the lender by falsifying applications to reach a desired score.
Typically the predictive power of an algorithm – the black box element of how it learns over time – comes at the expense of transparency and need for “explainable artificial intelligence (AI)”. There is also early evidence that discriminatory biases are less serious for fintech lenders than for traditional mortgage lenders in the US.
Alternative credit scoring presents heightened consent, privacy, and access issues
The opaque nature of machine learning-driven credit scoring is largely incompatible with the need for “informed consent” and the requirement for banks to be transparent about how they use data, where, and when. This is partly due to its experimental nature and its propensity to find new uses for data, and also because it may not fit contexts where data is observed rather than directly provided by data subjects.
The most useful data – whether it be customer location or enriched transaction data – has to be enabled or permissioned by the customer, and this permission isn’t always forthcoming in the absence of some clearly defined benefit (such as insights or offers). Banks will need to formalise an approach here, train staff to handle objections, and clearly explain privacy laws and the benefits of sharing data.
Data management risks
Meanwhile, global bank regulations around data security, customer privacy, and ethical use of data such as General Data Protection Regulation (GDPR) are making it increasingly challenging for financial institutions to share data among entities and across borders, to design target state data flows, and to develop meaningful analysis for the purpose of credit scoring. Complicating matters further, data generated by different activities is subject to different legal limitations on use. For example, data from a financial transaction cannot be used for the same purposes as the personal data from a profile on a social media platform.
This is an edited extract from the Alternative Credit Scoring – Thematic Research report produced by GlobalData Thematic Research.